By Yang Wenhao Editor: News Center Updated: Oct 28, 2020
Dr. Song Xiaoying, a young teacher of Professor Chai Li’s team has published a paper entitled Graph Signal Processing Approach to QSAR/QSPR Model Learning of Compounds (DOI: 10.1109/TPAMI.2020.3032718) on an international journal IEEE Transactions on Pattern Analysis and Machine Intelligence. This top journal in artificial intelligence has an impact factor of 17.86 and this is our first scientific research paper published in this journal.
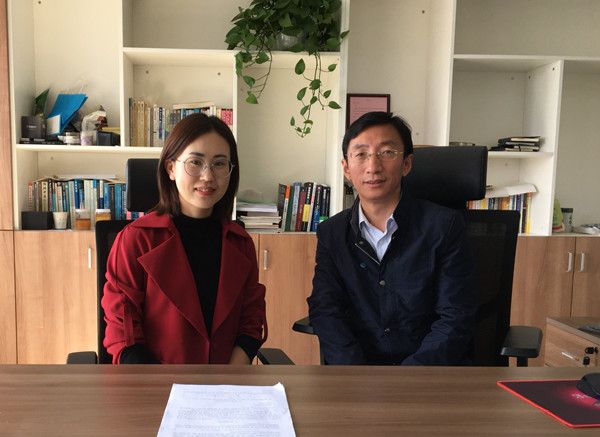
The first author of this paper is Dr. Song Xiaoying, a young teacher from our university, and the corresponding author is Professor Chai Li. Professor Zhang Jingxin from Swinburne University of Technology in Australia is the co-author. The first unit is our ministerial Engineering Research Center of Metallurgical Automation and Measurement Technology (the Ministry of Education).
IEEE T PAMI, a top international journal in artificial intelligence, received a 17.86 impact factor in 2019.
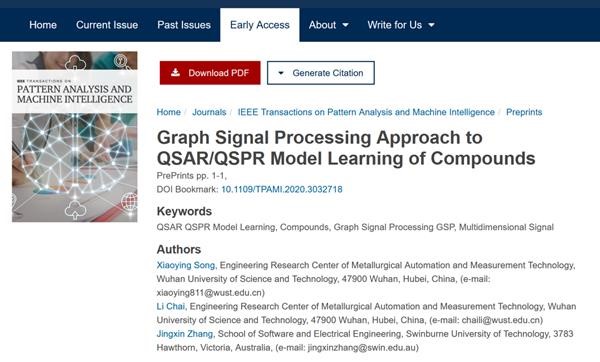
Screenshot of the Paper Acceptance
The paper presents an innovative model learning framework for a quantitative relationship between the physicochemical/active properties of compounds and the compound structure based on graph signal processing (QSAR/QSPR), which hence addressesthe bottleneck thatexisting methods cannot distinguish compounds with the same graphical structure and provides a significantly more predictive and stable algorithms. In this paper, a multidimensional signal for each vertex of the molecular graph is initiatively designed too to derive new descriptors with higher discriminability. Combining these with model learning techniques, a new molecular descriptor graph structure has been developed with enhanced descriptor dissimilarity using the Laplacian filter derived from the descriptor graph. As a result, a better performing and more robust nonlinear QSAR/QSPR model has been established.
This research is the first attempt to study QSAR/QSPR modeling of compounds from the perspective of graph signal processing and machine learning, providing a new AI-based method for compound prediction and classification. It has great scientific significance for crossover studies in graph learning and compound structure analysis.
Dr. Song Xiaoying, the first writer of this paper, and Professor Chai Li, the corresponding author both are from Engineering Research Center of Metallurgical Automation and Measurement Technology, Wuhan University of Science and Technology. And simultaneously Professor Zhang Jingxin, Swinburne University of Technology in Australia serves as the co-author.
.